Retention and Attrition in U.S. STEM Education with the Help of Computer Technology and Curriculum Development
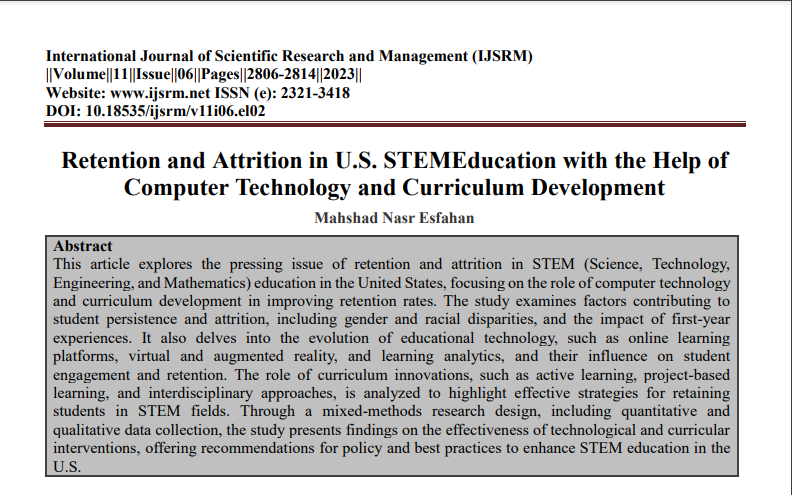
Submission to VIJ 2023-06-29
Keywords
- STEM retention, attrition, educational technology, curriculum development, active learning, project-based learning, interdisciplinary STEM education
Copyright (c) 2024 Mahshad Nasr Esfahan

This work is licensed under a Creative Commons Attribution 4.0 International License.
Abstract
This article explores the pressing issue of retention and attrition in STEM (Science, Technology, Engineering, and Mathematics) education in the United States, focusing on the role of computer technology and curriculum development in improving retention rates. The study examines factors contributing to student persistence and attrition, including gender and racial disparities, and the impact of first-year experiences. It also delves into the evolution of educational technology, such as online learning platforms, virtual and augmented reality, and learning analytics, and their influence on student engagement and retention. The role of curriculum innovations, such as active learning, project-based learning, and interdisciplinary approaches, is analyzed to highlight effective strategies for retaining students in STEM fields. Through a mixed-methods research design, including quantitative and qualitative data collection, the study presents findings on the effectiveness of technological and curricular interventions, offering recommendations for policy and best practices to enhance STEM education in the U.S.
References
- Bhadani, U. (2023, June). Verizon Telecommunication Network in Boston. In 2023 5th International Conference on Computer Communication and the Internet (ICCCI) (pp. 190-199). IEEE.
- Bhadani, U. (2020). Hybrid Cloud: The New Generation of Indian Education Society.
- Bhadani, U. (2024). Pillars of Power System and Security of Smart Grid. International Journal of Innovative Research in Science Engineering and Technology, 13(13888), 10-15680.
- Bhadani, U. (2024). Smart Grids: A Cyber–Physical Systems Perspective. International Research Journal of Engineering and Technology (IRJET), 11(06), 801.
- Leng, Q., & Peng, L. Medical Image Intelligent Diagnosis System Based on Facial Emotion Recognition and Convolutional Neural Network.
- Li, S., Lin, J., Shi, H., Zhang, J., Wang, S., Yao, Y., ... & Yang, K. (2024). DTCLMapper: Dual Temporal Consistent Learning for Vectorized HD Map Construction. arXiv preprint arXiv:2405.05518.
- Huang, S., Yang, H., Yao, Y., Lin, X., & Tu, Y. (2024). Deep Adaptive Interest Network: Personalized Recommendation with Context-Aware Learning. arXiv preprint arXiv:2409.02425.
- Yoosefinejad, A. K., & Ghalamghash, R. (2014). The Evaluation and prevalence of foot problems among Iranian students using" alfoots" company scanner. Health Science Journal, 8(3), 393.
- Ghalamghash, R., Gushe, B., & Omrani, A. (2008). keihani M, Fallahi A. The effect of cardiac rehabilitation on functional capabilities of patients with valvular heart surgery. Journal of Medical Council of Islamic Republic of Iran, 26(2), 213-21.
- Ghalamghash, R., Goosheh, B., Emrani, A., Keyhani, M. R., & Hosseini, A. (2007). Effects of cardiac rehabilitation programs on functional capacity following valvular heart surgery. Journal of Cardiopulmonary Rehabilitation and Prevention, 27(5), 346.
- Ghalamghash, R., Keyhani, M. R., Gousheh, B., BAZR, A. A., Barzegari, M., & Hoseini, A. (2006). The importance of phase I cardiac rehabilitation and evaluation of its performance in Tehran city hospitals.
- Bhat, N. P. (2023). Analysis of Safety Stock Determination Methodology-Quantity Vs. Time Buffers. Asia-Pacific Journal of Science and Technology, 28(06).