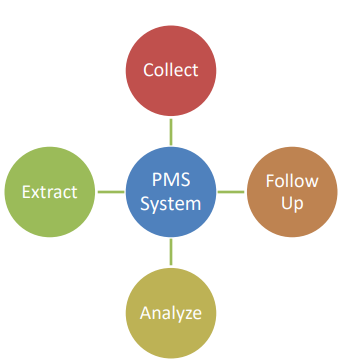
Submission to VIJ 2024-08-18
Keywords
- Artificial Intelligence ,
- Medical Devices
Copyright (c) 2024 Samadrita Ghosh

This work is licensed under a Creative Commons Attribution 4.0 International License.
Abstract
Artificial intelligence (AI) has emerged as a transformative tool in post-market surveillance (PMS) for monitoring the safety and performance of medical products. This article explores the role of AI in optimizing PMS practices, focusing on its applications in signal detection, risk assessment, and regulatory compliance. By harnessing machine learning algorithms and big data analytics, AI facilitates the automated analysis of real-world evidence, including patient outcomes data, adverse event reports, and electronic health records. Through pattern recognition and anomaly detection, AI algorithms enable the early identification of potential safety issues and facilitate timely interventions to mitigate risks. Moreover, AI-driven PMS systems enhance regulatory oversight by providing regulators with comprehensive and actionable insights into product safety profiles and emerging trends. However, concerns regarding data privacy, algorithm bias, and interpretability underscore the need for transparent and ethically responsible AI deployment in PMS frameworks.
References
- Watari R, Matsuda A, Ohnishi S, Hasegawa H (2019) Minimal contribution of P-gp on the low brain distribution of naldemedine, a peripherally acting μ-opioid receptor antagonist. Drug Metab Pharmacokinet 34(2):126–133. https://doi.org/10.1016/j.dmpk.2018.12.002.doi:10.1016/j.dmpk.2018.12.002.
- Coluzzi, F., M.S. Scerpa, and J. Pergolizzi, Naldemedine: A New Option for OIBD. J Pain Res, 2020. 13.
- Farmer, A.D., et al., Pathophysiology and management of opioid-induced constipation: European expert consensus statement. United European Gastroenterol J, 2019. 7.
- Hale, M., et al., Naldemedine versus placebo for opioid-induced constipation (COMPOSE-1 and COMPOSE-2): two multicentre, Phase 3, double-blind, randomised, parallel-group trials. Lancet Gastroenterol Hepatol, 2017. 2.
- Kanemasa, T., et al., Pharmacologic effects of naldemedine, a peripherally acting μ-opioid receptor antagonist, in in vitro and in vivo models of opioid-induced constipation. Neurogastroenterol Motil., 2019. 31.
- Katakami, N., et al., Randomized Phase III and Extension Studies of Naldemedine in Patients With Opioid-Induced Constipation and Cancer. J Clin Oncol, 2017. 3.
- Katakami, N., et al., Randomized phase III and extension studies: efficacy and impacts on quality of life of naldemedine in subjects with opioid-induced constipation and cancer. Ann Oncol, 2018. 29.
- Katakami, N., et al., Phase IIb, Randomized, Double-Blind, Placebo-Controlled Study of Naldemedine for the Treatment of Opioid-Induced Constipation in Patients with Cancer. J Clin Oncol, 2017. 35.
- Saito, Y., et al., Naldemedine in Japanese patients with opioid-induced constipation and chronic noncancer pain: open-label phase III studies. J Pain Res, 2019. 12.
- Webster, L.R., T. Yamada, and J.C. Arjona Ferreira, A phase 2b, randomized, double-blind placebo-controlled study to evaluate the efficacy and safety of naldemedine for the treatment of opioid-induced constipation in patients with chronic noncancer pain. Pain Med, 2017. 18.
- Viscusi, E.R., Clinical Overview and Considerations for the Management of Opioid-induced Constipation in Patients With Chronic Noncancer Pain. Clin J Pain, 2019. 35.
- Viscusi, E.R. and A.R. Viscusi, Blood-brain barrier: mechanisms governing permeability and interaction with peripherally acting μ-opioid receptor antagonists. Reg Anesth Pain Med, 2020. 45.
- Webster, L.R., et al., Long-term use of naldemedine in the treatment of opioid-induced constipation in patients with chronic noncancer pain: a randomized, double-blind, placebo-controlled phase 3 study. Pain, 2018. 159.
- Brahnam, S., Jain, L.C. (eds.): Advanced Computational Intelligence Paradigms in Healthcare 6: Virtual Reality in Psychotherapy, Rehabilitation, and Assessment. Springer, Heidelberg (2011).
- Bichindaritz, I.S., et al. (eds.): Advanced Computational Intelligence Paradigms in Healthcare 4: Advanced Methodologies. Springer, Heidelberg (2010).
- Brahnam, S., Jain, L.C. (eds.): Advanced Computational Intelligence Paradigms in Healthcare 5: Intelligent Decision Support Systems. Springer, Heidelberg (2010).
- Vaidya, S., et al. (eds.): Advanced Computational Intelligence Paradigms in Healthcare 2. Springer, Heidelberg (2008).
- Sardo, M., et al. (eds.): Advanced Computational Intelligence Paradigms in Healthcare 3. Springer, Heidelberg (2008).
- Yoshida, H., et al. (eds.): Advanced Computational Intelligence Paradigms in Healthcare 1. Springer, Heidelberg (2007).
- Ichalkaranje, N., et al. (eds.): Intelligent Paradigms for Assistive and Preventive Healthcare. Springer, Heidelberg (2006).
- Silverman, B., et al. (eds.): Intelligent Paradigms in Healthcare Enterprises. Springer, Heidelberg (2005).
- Jain, A., et al. (eds.): Artificial Intelligence Techniques in Breast Cancer Diagnosis and Prognosis. World Scientific (2000).
- Chen, Y., et al. (eds.): Innovation in medicine and healthcare 2021. In: Proceedings of the KES-InMed 2021 Conference. Springer, Germany (2021).
- Chen, Y., et al. (eds.): Innovation in medicine and healthcare 2022. In: Proceedings of the KES-InMed 2022 Conference. Springer, Germany (2022).
- Chen, et al. (eds.): Innovation in medicine and healthcare 2017. In: Proceedings of the KES-InMed 2017 Conference. Springer, Germany (2017).
- Badnjevic, A., Evidence-based maintenance of medical devices: current shortage and pathway towards solution. Technol Health Care, 2023. 31.
- Chen, Y., et al. (eds.): Innovation in medicine and healthcare 2016. In: Proceedings of the KES-InMed 2016 Conference. Springer, Germany (2016).
- Chen, Y., et al. (eds.): Innovation in medicine and healthcare 2015. In Proceedings of the KES-InMed 2015 Conference. Springer, Germany (2015).
- Grana, M., et al. (eds.): Innovation in Medicine and Healthcare. IOS Press (2014).
- Holsapple, C., Whinston, A., Whinston, A.: Bussiness Expert Systems. McGraw-Hill (1987).
- Leondes, C.T.: Expert Systems: The Technology of Knowledge Management and Decision Making for the 21st Century, pp. 1–22 (2002).
- Xu, L., Jiang, L., Qin, C., Wang, Z., Du, D.: How images inspire poems: generating classical Chinese poetry from images with memory networks. In: Proceedings of the Thirty-Second AAAI Conference on Artificial Intelligence and Thirtieth Innovative Applications of Artificial Intelligence Conference and Eight AAAI Symposium on Educational Advances in Artificial Intelligence, vol. 689, pp. 5618–5625 (2018).
- Shortliffe, E.: Computer-Based Medical Consultations: MYCIN. Elsevier (1976).
- Belciug, S., Gorunescu, F.: Era of intelligent systems in healthcare. In: Belciug, S., Gorunescu, F. (eds.) Intelligent Decision Support Systems: A Journey to Smarter Healthcare. Springer, Heidelberg (2020).
- Sim, I., Gorman, P., Greenes, R.A., et al.: Clinical decision support systems for the practice of evidence-based medicine. J. Am. Med. Inform, Assoc. 8(6), 527–534 (2001).
- Basilico, J. (2019). Netflix research. Retrieved from https://research.netflix.com/research-area/machine-learning.
- Blackwood, A. (2021). Data visualisation: Basics & trends. Retrieved from https://blog.iotechnologies.com/data-visualization-trends.
- Buuck, B. (2022). The operational data store (ODS) vs data warehouse. Retrieved from https://streamsets.com/blog/operational-data-stores-ods-data-warehouses.
- Clayton, T. (2019). The difference between implicit and explicit data for business. Retrieved from https://blog.mirumee.com/the-difference-between-implicit-and-explicit-data-for-business-351f70ff3fbf.
- Cubeware Gmbh. (2022). Structured data: Definition, examples, and comparison. Retrieved from https://www.linkedin.com/pulse/structured-data-definition-examples-comparison-cubeware-gmbh.
- CX Trend. (2022). The Zendesk customer experience trends report 2022. Retrieved from https://www.zendesk.com/customer-experience-trends.
- David, D. (2021). AI vs ML – What’s the difference between artificial intelligence and machine learning? Retrieved from https://www.freecodecamp.org/news/ai-vs-ml-whats-the-difference.
- Grieve, P. (2022). The importance of providing personalized service in 2022. Retrieved from https://www.zendesk.com/in/blog/start-providing-personalized-customer-service/#georedirect.
- Han, M. (2021). Analysis of the public’s intention to use the government's artificial intelligence (AI)-based services: Focusing on public values and extended technology acceptance model. The Journal of the Korea Contents Association, 21(8), 388–402.
- Hyken, S. (2017). Personalized customer experience increases revenue and loyalty. Retrieved from https://www.forbes.com/sites/shephyken/2017/10/29/personalized-customer-experience-increases-revenue-and-loyalty/?sh=62f148054bd6.
- Hyperight. (2021). Deep brew: Transforming Starbucks into an AI & data-driven company. Hyperight.com. https://hyperight.com/deep-brew-transforming-starbucks-into-a-data-driven-company.
- IBM. (2021). Structured vs. unstructured data: What’s the difference? Retrieved from https://www.ibm.com/cloud/blog/structured-vs-unstructured-data.
- IBM Cloud Education. (2021). What is data visualization? Retrieved from https://www.ibm.com/cloud/learn/data-visualization.
- Kostusev, D. (2018). Personalization in sales: Why it matters and how to achieve it. Retrieved from https://www.forbes.com/sites/forbesbusinessdevelopmentcouncil/2018/06/11/personalization-in-sales-why-it-matters-and-how-to-achieve-it/?sh=5a0ffd819127.
- Kotsiantis, S. (2006). Association rules mining: A recent overview. Retrieved from https://citeseerx.ist.psu.edu/viewdoc/download?doi=10.1.1.103.6295&rep=rep1&type=pdf.
- Krishnan, S., Franklin, M. J., Goldberg, K., Wang, J., & Wu, E. (2016). Active clean: An interactive data cleaning framework for modern machine learning. In Proceedings of the 2016 international conference on management of data. https://doi.org/10.1145/2882903.2899409.
- Kumar, V., & Khosla, C. (2018). Data Cleaning-A thorough analysis and survey on unstructured data. In 2018 8th international conference on cloud computing, data science & engineering (Confluence).https://doi.org/10.1109/confluence.2018.8442950.
- Lenzerini, M. (2002). Data integration. Proceedings of the twenty-first ACM SIGMOD-SIGACT-SIGART symposium on principles of database systems - PODS '02. https://doi.org/10.1145/543613.543644.
- Chaudhry, H. A. H., Renzulli, R., Perlo, D., Santinelli, F., Tibaldi, S., Cristiano, C., Grosso, M., Limerutti, G., Fiandrotti, A., Grangetto, M., et al. (2022). UniToChest: A lung image dataset for segmentation of cancerous nodules on CT scans. In: Image analysis and processing–ICIAP 2022: 21st international conference, Lecce, Italy, May 23–27, 2022, Proceedings, Part I (pp. 185–196). Springer.
- De Streel, A., Bibal, A., Frénay, B., Lognoul, M. (2020). Explaining the black box: When law controls AI. CERRE.
- Tietjen, D., von Woedtke, N., Schwind, E. (2021). Artificial intelligence act (AIA)—Legal uncertainty for medical device manufacturers.
- Badnjević, A., L.G. Pokvić, and Z. Džemić, Risks of emergency use authorizations for medical products during outbreak situations: a COVID-19 case study. BioMed Eng OnLine, 2020. 19.
- Amantea, I.A., et al., Adopting assistive technologies in healthcare processes: A chatbot for patients with amyotrophic lateral sclerosis, in Italian forum of ambient assisted living. 2022, Springer.
- Amantea, I.A., et al., A process mining application for the analysis of hospital-at-home admissions. Studies in Health Technology and Informatics, 2020. 270.
- Ebers, M., Standardizing AI-the case of the European commission’s proposal for an artificial intelligence act, in The Cambridge handbook of artificial intelligence: Global perspectives on law and ethics. 2021, Cambridge University Press.
- Ebers, M., et al., The European commission’s proposal for an artificial intelligence act—A critical assessment by members of the robotics and AI law society (RAILS). Multidisciplinary Scientific Journal, 2021. 4.
- Khambati, A. (2021). Innovative Smart Water Management System Using Artificial Intelligence. Turkish Journal of Computer and Mathematics Education (TURCOMAT), 12(3), 4726-4734.
- JOSHI, D., SAYED, F., BERI, J., & PAL, R. (2021). An efficient supervised machine learning model approach for forecasting of renewable energy to tackle climate change. Int J Comp Sci Eng Inform Technol Res, 11, 25-32.
- Khambaty, A., Joshi, D., Sayed, F., Pinto, K., & Karamchandani, S. (2022, January). Delve into the Realms with 3D Forms: Visualization System Aid Design in an IOT-Driven World. In Proceedings of International Conference on Wireless Communication: ICWiCom 2021 (pp. 335-343). Singapore: Springer Nature Singapore.
- Joshi, D., Sayed, F., Jain, H., Beri, J., Bandi, Y., & Karamchandani, S. A Cloud Native Machine Learning based Approach for Detection and Impact of Cyclone and Hurricanes on Coastal Areas of Pacific and Atlantic Ocean.
- Joshi, D., Sayed, F., Saraf, A., Sutaria, A., & Karamchandani, S. (2021). Elements of Nature Optimized into Smart Energy Grids using Machine Learning. Design Engineering, 1886-1892.
- Joshi, D., Parikh, A., Mangla, R., Sayed, F., & Karamchandani, S. H. (2021). AI Based Nose for Trace of Churn in Assessment of Captive Customers. Turkish Online Journal of Qualitative Inquiry, 12(6).
- JALA, S., ADHIA, N., KOTHARI, M., JOSHI, D., & PAL, R. SUPPLY CHAIN DEMAND FORECASTING USING APPLIED MACHINE LEARNING AND FEATURE ENGINEERING.
- Floridi, L., et al., AI4People—An ethical framework for a good AI society: Opportunities, risks, principles, and recommendations. Minds and Machines, 2018. 28.
- Jin, Y.H., et al., A rapid advice guideline for the diagnosis and treatment of 2019 novel coronavirus (2019-nCoV) infected pneumonia (standard version). Military Medical Research, 2020. 7.
- Marchi, F., et al., E-health solutions for amyotrophic lateral sclerosis patients: A chatbot for dietary monitoring. Journal of the Neurological Sciences, 2021. 429.
- Minssen, T., M. Mimler, and V. Mak, When does stand-alone software qualify as a medical device in the European Union?—The CJEU’s decision in Snitem and what it implies for the next generation of medical devices. Medical Law Review, 2020. 28.
- Munoz-Gama, J., et al., Process mining for healthcare: Characteristics and challenges. Journal of Biomedical Informatics, 2022. 127.
- Palmieri, S., P. Walraet, and T. Goffin, Inevitable influences: AI-based medical devices at the intersection of medical devices regulation and the proposal for AI regulation. European Journal of Health Law, 2021. 28.
- Veale, M. and F.Z. Borgesius, Demystifying the draft EU artificial intelligence act-analysing the good, the bad, and the unclear elements of the proposed approach. Computer Law Review International, 2021. 22.
- Zhang, X., et al., Prediction of emergency department hospital admission based on natural language processing and neural networks. Methods of Information in Medicine, 2017. 56.
- Senbekov M et al (2020) The recent progress and applications of digital technologies in healthcare: a review. Int J Telemed Appl.
- Effective post-market surveillance: Understanding and conducting vigilance and post-market clinical follow-up [Pamphlet]. BSI Standards Ltd., London (UK) (2014).
- Food and Drug Administration (FDA) [Internet]. Food and Drug Administration, Silver Spring (MA).
- EUDAMED Database. https://ec.europa.eu/tools/eudamed/eudamed.
- MEDSun. https://www.fda.gov/medical-devices/medical-device-safety/medsun-medical-product-safety-network.
- Badnjević A et al (2022) Post-market surveillance of medical devices: a review. Technol Health Care 30(6):1315–1329.
- Badnjević A, Pokvić LG, Hasičić M, Bandić L, Mašetić Z, Kovačević Ž, Kevrić J, Pecchia L (2019) Evidence-based clinical engineering: machine learning algorithms for prediction of defibrillator performance. Biomed Sig Process Control 54:101629.