Emerging Technology Integration - Artificial Intelligence (AI) and Machine Learning (ML) for Predictive Analysis for Safety and Toxicity Assessment in Environmental Toxicology
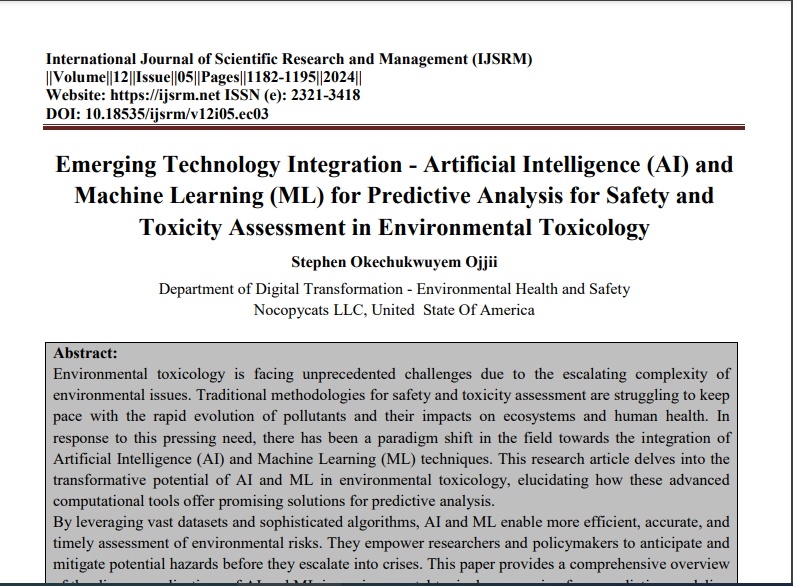
Submission to VIJ 2024-05-11
Keywords
- Artificial Intelligence, Machine Learning, Environmental Toxicology, Predictive Analysis, Safety Assessment, Toxicity Assessmental
Copyright (c) 2024 Stephen Okechukwuyem Ojji

This work is licensed under a Creative Commons Attribution 4.0 International License.
Abstract
Environmental toxicology is facing unprecedented challenges due to the escalating complexity of environmental issues. Traditional methodologies for safety and toxicity assessment are struggling to keep pace with the rapid evolution of pollutants and their impacts on ecosystems and human health. In response to this pressing need, there has been a paradigm shift in the field towards the integration of Artificial Intelligence (AI) and Machine Learning (ML) techniques. This research article delves into the transformative potential of AI and ML in environmental toxicology, elucidating how these advanced computational tools offer promising solutions for predictive analysis.
By leveraging vast datasets and sophisticated algorithms, AI and ML enable more efficient, accurate, and timely assessment of environmental risks. They empower researchers and policymakers to anticipate and mitigate potential hazards before they escalate into crises. This paper provides a comprehensive overview of the diverse applications of AI and ML in environmental toxicology, ranging from predictive modeling of chemical hazards to early detection of environmental threats.
Furthermore, it highlights the potential of AI and ML to revolutionize decision-making processes in environmental management and policy formulation. By synthesizing vast amounts of data and identifying complex patterns and correlations, these technologies offer invaluable insights for crafting evidence-based policies and strategies to safeguard the environment and public health.
This research article underscores the pivotal role of AI and ML in addressing the multifaceted challenges of environmental toxicology. Through their integration, we have the opportunity to enhance our understanding of environmental risks, optimize resource allocation, and ultimately, pave the way towards a more sustainable and resilient future.
References
- Miller, T. H., Gallidabino, M. D., MacRae, J. I., Hogstrand, C., Bury, N. R., Barron, L. P., ... & Owen, S. F. (2018). Machine learning for environmental toxicology: a call for integration and innovation.
- Oyeniyi, J., & Oluwaseyi, P. Emerging Trends in AI-Powered Medical Imaging: Enhancing Diagnostic Accuracy and Treatment Decisions.
- Singh, A. V., Rosenkranz, D., Ansari, M. H. D., Singh, R., Kanase, A., Singh, S. P., ... & Luch, A. (2020). Artificial intelligence and machine learning empower advanced biomedical material design to toxicity prediction. Advanced Intelligent Systems, 2(12), 2000084.
- Rehan, H. (2024). The Future of Electric Vehicles: Navigating the Intersection of AI, Cloud Technology, and Cybersecurity. Valley International Journal Digital Library, 1127-1143.
- Jiao, Z., Hu, P., Xu, H., & Wang, Q. (2020). Machine learning and deep learning in chemical health and safety: a systematic review of techniques and applications. ACS Chemical Health & Safety, 27(6), 316-334.
- Chadee, A. A., Martin, H. H., Mwasha, A., & Otuloge, F. (2022). Rationalizing critical cost overrun factors on public sector housing programmes. Emerging Science Journal, 6(3), 647-666.
- Singh, A. V., Ansari, M. H. D., Rosenkranz, D., Maharjan, R. S., Kriegel, F. L., Gandhi, K., ... & Luch, A. (2020). Artificial intelligence and machine learning in computational nanotoxicology: unlocking and empowering nanomedicine. Advanced Healthcare Materials, 9(17), 1901862.
- Rehan, Hassan. "AI-Driven Cloud Security: The Future of Safeguarding Sensitive Data in the Digital Age." Journal of Artificial Intelligence General science (JAIGS) ISSN: 3006-4023 1, no. 1 (2024): 47-66.
- Zhong, S., Zhang, K., Bagheri, M., Burken, J. G., Gu, A., Li, B., ... & Zhang, H. (2021). Machine learning: new ideas and tools in environmental science and engineering. Environmental Science & Technology, 55(19), 12741-12754.
- Singh, A. V., Varma, M., Laux, P., Choudhary, S., Datusalia, A. K., Gupta, N., ... & Nath, B. (2023). Artificial intelligence and machine learning disciplines with the potential to improve the nanotoxicology and nanomedicine fields: a comprehensive review. Archives of toxicology, 97(4), 963-979.
- Pulicharla, M. R. (2023). Hybrid Quantum-Classical Machine Learning Models: Powering the Future of AI. Journal of Science & Technology, 4(1), 40-65.
- Mittal, C. (2024). An Empirical Study on Cybersecurity Awareness, Cybersecurity Concern, and Vulnerability to Cyber-attacks. Valley International Journal Digital Library, 1144-1158.
- Oyeniyi, J. UNVEILING THE COGNITIVE CAPACITY OF CHATGPT: ASSESSING ITS HUMAN-LIKE REASONING ABILITIES.
- Wang, X., Wu, Y. C., & Ma, Z. (2024). Blockchain in the courtroom: exploring its evidentiary significance and procedural implications in US judicial processes. Frontiers in Blockchain, 7, 1306058.
- Ramirez, J. G. C. (2024). Transversal Threats and Collateral Conflicts: Communities of the United States under the siege of political conflicts on the American continent. International Journal of Culture and Education, 2(1).
- Sarker, M. (2022). Towards Precision Medicine for Cancer Patient Stratification by Classifying Cancer By Using Machine Learning. Journal of Science & Technology, 3(3), 1-30.
- Pulicharla, M. R. (2024). Data Versioning and Its Impact on Machine Learning Models. Journal of Science & Technology, 5(1), 22-37.
- Guo, H., Ma, Z., Chen, X., Wang, X., Xu, J., & Zheng, Y. (2024). Generating Artistic Portraits from Face Photos with Feature Disentanglement and Reconstruction. Electronics, 13(5), 955.
- Adeyeri, T. B. (2024). Enhancing Financial Analysis Through Artificial Intelligence: A Comprehensive Review. Journal of Science & Technology, 5(2), 102-120.
- Kohil, A., Amir, S. S., Behrens, A., & Khan, O. M. (2023). A small Rho GTPase RAB25 with a potential role in chemotherapy resistance in pancreatic cancer. Cancer Biomarkers, 36(2), 133-145.
- Asaju, B. J. (2024). Privacy Preservation Techniques in V2X Ecosystems: Safeguarding Individual Privacy in Connected Vehicle Environments. Journal of Artificial Intelligence Research, 4(1), 58-72.
- Rotolo, D., Hicks, D., & Martin, B. R. (2015). What is an emerging technology?. Research policy, 44(10), 1827-1843.
- Weng, Y., & Wu, J. (2024). Fortifying the global data fortress: a multidimensional examination of cyber security indexes and data protection measures across 193 nations. International Journal of Frontiers in Engineering Technology, 6(2), 13-28.
- Ertmer, P. A. (1999). Addressing first-and second-order barriers to change: Strategies for technology integration. Educational technology research and development, 47(4), 47-61.
- Keyan, K. S., Alanany, R., Kohil, A., & Khan, O. M. (2023). E3 Ubiquitin Ligase TRIP12 controls exit from mitosis via positive regulation of MCL-1 in response to taxol. Cancers, 15(2), 505.
- Kimmons, R., & Hall, C. (2016). Emerging technology integration models. Foundations and Applications.
- Oyeniyi, J. UNVEILING THE COGNITIVE CAPACITY OF CHATGPT: ASSESSING ITS HUMAN-LIKE REASONING ABILITIES.
- Asaju, B. J. (2024). Advancements in Intrusion Detection Systems for V2X: Leveraging AI and ML for Real-Time Cyber Threat Mitigation. Journal of Computational Intelligence and Robotics, 4(1), 33-50.
- Shen, Y. C., Chang, S. H., Lin, G. T., & Yu, H. C. (2010). A hybrid selection model for emerging technology. Technological Forecasting and Social Change, 77(1), 151-166.
- Li, X., Wang, X., Chen, X., Lu, Y., Fu, H., & Wu, Y. C. (2024). Unlabeled data selection for active learning in image classification. Scientific Reports, 14(1), 424.
- Heston, T. F., & Pahang, J. A. (2019). Moral injury and the four pillars of bioethics. F1000Research, 8.
- Jordan, M. I., & Mitchell, T. M. (2015). Machine learning: Trends, perspectives, and prospects. Science, 349(6245), 255-260.
- Liang, Y., Wang, X., Wu, Y. C., Fu, H., & Zhou, M. (2023). A Study on Blockchain Sandwich Attack Strategies Based on Mechanism Design Game Theory. Electronics, 12(21), 4417.
- Adeyeri, T. B. (2024). Automating Accounting Processes: How AI is Streamlining Financial Reporting. Journal of Artificial Intelligence Research, 4(1), 72-90.
- Sammut, C., & Webb, G. I. (Eds.). (2011). Encyclopedia of machine learning. Springer Science & Business Media.
- Harrington, P. (2012). Machine learning in action. Simon and Schuster.
- Rehan, H. (2024). AI-Driven Cloud Security: The Future of Safeguarding Sensitive Data in the Digital Age. Journal of Artificial Intelligence General science (JAIGS) ISSN: 3006-4023, 1(1), 47-66.
- Sharifani, K., & Amini, M. (2023). Machine learning and deep learning: A review of methods and applications. World Information Technology and Engineering Journal, 10(07), 3897-3904.
- Li, W., Wang, C. H., Cheng, G., & Song, Q. (2023). International conference on machine learning. Transactions on machine learning research.
- Adeyeri, T. B. (2024). Blockchain and AI Synergy: Transforming Financial Transactions and Auditing. Blockchain Technology and Distributed Systems, 4(1), 24-44.
- Lee, Z., Wu, Y. C., & Wang, X. (2023, October). Automated Machine Learning in Waste Classification: A Revolutionary Approach to Efficiency and Accuracy. In Proceedings of the 2023 12th International Conference on Computing and Pattern Recognition (pp. 299-303).