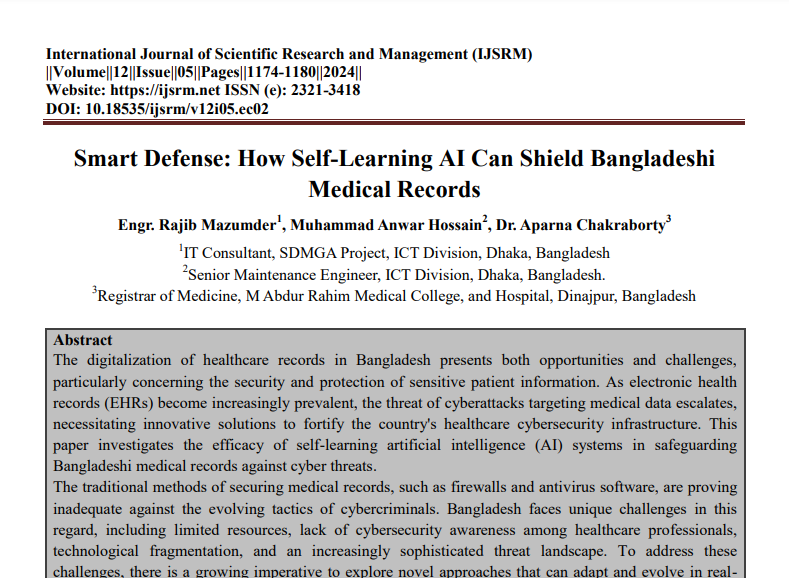
Submission to VIJ 2024-05-08
Keywords
- Self-Learning AI
Copyright (c) 2024 Engr. Rajib Mazumder, Muhammad Anwar Hossain, Dr. Aparna Chakraborty

This work is licensed under a Creative Commons Attribution 4.0 International License.
Abstract
The digitalization of healthcare records in Bangladesh presents both opportunities and challenges, particularly concerning the security and protection of sensitive patient information. As electronic health records (EHRs) become increasingly prevalent, the threat of cyberattacks targeting medical data escalates, necessitating innovative solutions to fortify the country's healthcare cybersecurity infrastructure. This paper investigates the efficacy of self-learning artificial intelligence (AI) systems in safeguarding Bangladeshi medical records against cyber threats.
The traditional methods of securing medical records, such as firewalls and antivirus software, are proving inadequate against the evolving tactics of cybercriminals. Bangladesh faces unique challenges in this regard, including limited resources, lack of cybersecurity awareness among healthcare professionals, technological fragmentation, and an increasingly sophisticated threat landscape. To address these challenges, there is a growing imperative to explore novel approaches that can adapt and evolve in real-time to counter emerging cyber threats.
Self-learning AI systems represent a promising frontier in healthcare cybersecurity. By leveraging advanced machine learning algorithms, these systems can analyze vast amounts of data to detect patterns indicative of cyber threats. Unlike static security measures, self-learning AI continuously learns from new information and adjust their defense strategies, accordingly, enabling them to stay ahead of evolving threats. Key functionalities of self-learning AI include anomaly detection, threat prediction, and adaptive defense mechanisms, all of which are essential for safeguarding medical records in Bangladesh's healthcare landscape.
The implications of integrating self-learning AI into Bangladesh's healthcare cybersecurity framework are significant. Not only can these technologies enhance the detection and prevention of cyber threats, but they can also alleviate resource constraints and technical challenges faced by healthcare organizations. However, successful implementation requires comprehensive training, adherence to data privacy regulations, and ongoing monitoring to ensure the effectiveness and reliability of AI-driven security measures.
The protection of medical records is paramount as Bangladesh continues its digital transformation in healthcare. Self-learning AI offers a dynamic and proactive approach to cybersecurity, empowering healthcare organizations to mitigate risks and preserve patient privacy in an increasingly digitized landscape. Embracing these innovative technologies is crucial for building a resilient healthcare ecosystem that prioritizes data security and patient trust.
References
- Alabdulatif, A., Khalil, I., & Saidur Rahman, M. (2022). Security of blockchain and AI-empowered smart healthcare: Application-based analysis. Applied Sciences, 12(21), 11039.
- Yu, S., & Lu, Y. (2021). An introduction to artificial intelligence in education. Springer.
- Mandal, J. K., Misra, S., Banerjee, J. S., & Nayak, S. (Eds.). (2022). Applications of Machine Intelligence in Engineering: Proceedings of 2nd Global Conference on Artificial Intelligence and Applications (GCAIA, 2021), September 8-10, 2021, Jaipur, India. CRC Press.
- Nair, C. D. J. N. (Ed.). (2023). Emerging Defence, Maritime and Aerospace Technologies by DRaS. Highlyy Publishing LLP.
- Debnath, O., Debnath, S., Karmakar, S., Mallick, M. T., & Saha, H. N. A Novel IoT Architecture, Assessment of Threats, and Their Classification with Machine Learning Solutions.
- Esposito, M., & Kapoor, A. (2022). the Emerging Economies under the dome of the Fourth industrial revolution. Cambridge University Press.
- Puaschunder, J. (2022). Artificial Intelligence and Nudging. In Advances in Behavioral Economics and Finance Leadership: Strategic Leadership, Wise Followership and Conscientious Usership in the Digital Century (pp. 133-196). Cham: Springer International Publishing.
- Hasan, M. M. (2021). Distributed denial of service attack detection in cloud computing environment using machine learning.
- Abie, H., Gkotsis, I., Athanatos, M., Ugarelli, R. M., Čaleta, D., Lodi, L., ... & Jovanović, A. (2023). Consolidated Proceedings of the Second ECSCI Workshop on Critical Infrastructure Protection and Resilience. Steinbeis-Edition.
- Adeyeri, T. B. (2024). Automating Accounting Processes: How AI is Streamlining Financial Reporting. Journal of Artificial Intelligence Research, 4(1), 72-90.
- Lyon, D. (2021). Pandemic surveillance. John Wiley & Sons.
- Chertoff, M. (2018). Exploding Data: Reclaiming Our Cyber Security in the Digital Age. Atlantic Books.
- Shetty, M., Habib, F., Imran Ali, S., Haq, A., & Khan, M. (2023). Impact of Digitalisation in Developing Procurement and Supply Chain Resilience in the Post Pandemic Era—A Study of the Global Manufacturing Sector. In Advanced Technologies and the Management of Disruptive Supply Chains: The Post-COVID Era (pp. 109-151). Cham: Springer Nature Switzerland.
- Avramidou, M., Biasin, E., Kamenjasevic, E., Kun, E., & Nisevic, M. (2023, February). Cybersecurity and the NIS2 Directive: regulatory aspects and sectoral perspectives. In Consolidated Proceedings of the Second ECSCI Workshop on Critical Infrastructure Protection and Resilience (pp. 91-92). Steinbeis-Edition.
- Adeyeri, T. B. (2024). Enhancing Financial Analysis Through Artificial Intelligence: A Comprehensive Review. Journal of Science & Technology, 5(2), 102-120.
- PAUL, M. DIP: 18.20. 71650381.022 VISUAL APPROACHES OF FESTIVITY IN INDIAN PRINT ADVERTISEMENTS DURING COVID-19. MULTIDISCIPLINARY SUBJECTS FOR RESEARCH-II, 1, 89.
- Dahl, K. E., & Lillebø, N. (2019). How can the emerging technologies make Norwegian foreign Aid more efficient? (Master's thesis, Nord universitet).
- LEARNING, S. (2013). An International Perspective on N ext-Generation Technology-Enhanced Learning Marcelo Milrad, Lung-Hsiang Wong, Mike Sharples, Gwo-Ien Hwang, Chee-Kit Looi, and Hiroaki Ogata. Handbook of Mobile Learning, 95.
- Trivedi, D. A., Bhattacharjee, S., Pandey, U. S., Gupta, S., Satyan, U., Rana, R., ... & Thakar, M. (2022). Liberal Studies: Vol. 7 No. 1 (2022), January-June 2022. IndraStra Global e-Journal Hosting Services.
- Ullrich, C. (2018). A risk-based approach towards infringement prevention on the internet: adopting the anti-money laundering framework to online platforms. International Journal of Law and Information Technology, 26(3), 226-251.
- Fasnacht, D., & Fasnacht, D. (2018). Disruptive Trends. Open Innovation Ecosystems: Creating New Value Constellations in Financial Services, 25-68.
- Bansal, A. K., Khan, J. I., & Alam, S. K. (2019). Introduction to computational health informatics. CRC Press.
- Adeyeri, T. B. (2024). Blockchain and AI Synergy: Transforming Financial Transactions and Auditing. Blockchain Technology and Distributed Systems, 4(1), 24-44.