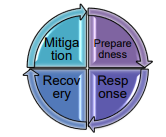
Submission to VIJ 2023-12-20
Keywords
- Prototype,
- Disaster Management,
- Flood Prediction,
- Internet of Things,
- Machine Learning
Copyright (c) 2023 Nur Laily, Juwita Sari, Muktar Redy Susila

This work is licensed under a Creative Commons Attribution 4.0 International License.
Abstract
This prototype output research has the aim of creating a dashboard that is used to monitor river water levels. The dashboard created will display data in real-time and prediction results. The use of the dashboard is to minimize the risk in the event of flooding caused by river overflow. The way this prototype works is to take data from sensors that have been installed at several points. The recorded data will be stored in a database using the working principles of the Internet of Things. For predictions, machine learning is used to produce future river water level figures. The machine learning used is using time series regression with rainfall input and river water level output. Long-term output data is needed, therefore to forecast future rainfall the Hybrid method is used. Data generated from sensors as well as from prediction results are stored in one database. From the database, data visualization is displayed along with important figures used for river overflow intelligence. Therefore, the dashboard is very useful for people living around the river flow.
References
- Andayani, M., Indrasari, W., & Iswanto, B. H. (2016). Kalibrasi Sensor Ultrasonik HC-SR04 sebagai Sensor Pendeteksi Jarak pada Prototipe Sistem Peringatan Dini Bencana Banjir. Prosiding Seminar Nasional Fisika (E-Journal), 5, 43–46.
- Andayani, M., Indrasari, W., & Iswanto, B. H. (2016). Kalibrasi Sensor Ultrasonik HC-SR04 sebagai Sensor Pendeteksi Jarak pada Prototipe Sistem Peringatan Dini Bencana Banjir. Prosiding Seminar Nasional Fisika (E-Journal), 5, 43–46.
- Bentivoglio, R., Isufi, E., Jonkman, S. N., & Taormina, R. (2022). Deep learning methods for flood mapping: a review of existing applications and future research directions. Hydrology and Earth System Sciences, 26(16), 4345–4378. https://doi.org/10.5194/hess-26-4345-2022
- Chang, L. C., Chang, F. J., Yang, S. N., Kao, I. F., Ku, Y. Y., Kuo, C. L., & Amin, I. M. Z. bin M. (2018). Building an intelligent hydroinformatics integration platform for regional flood inundation warning systems. Water (Switzerland), 11(1). https://doi.org/10.3390/w11010009
- Chang, L. C., Shen, H. Y., Wang, Y. F., Huang, J. Y., & Lin, Y. T. (2010). Clustering-based hybrid inundation model for forecasting flood inundation depths. Journal of Hydrology, 385(1–4), 257–268. https://doi.org/10.1016/j.jhydrol.2010.02.028
- Chidthong, Y., Tanaka, H., & Supharatid, S. (2009). Developing a hybrid multi-model for peak flood forecasting. Hydrological Processes, 2274(1 april 2009), 2267–2274. https://doi.org/10.1002/hyp
- Chitwatkulsiri, D., Miyamoto, H., Irvine, K. N., Pilailar, S., & Loc, H. H. (2022). Development and Application of a Real-Time Flood Forecasting System (RTFlood System) in a Tropical Urban Area: A Case Study of Ramkhamhaeng Polder, Bangkok, Thailand. Water, 14(1641).
- Kaur, M., Kaur, P. D., & Sood, S. K. (2021). Energy Efficient IoT Based Cloud Framework for Early Flood Prediction. Research Square. https://doi.org/10.1007/s11069-021-04910-7
- Munawar, H. S., Hammad, A. W. A., Waller, S. T., Thaheem, M. J., & Shrestha, A. (2021). An integrated approach for post-disaster flood management via the use of cutting-edge technologies and UAVs: A review. Sustainability (Switzerland), 13(14). https://doi.org/10.3390/su13147925
- Puttinaovarat, S., & Horkaew, P. (2020). Flood Forecasting System Based on Integrated Big and Crowdsource Data by Using Machine Learning Techniques. IEEE Access, 8, 5885–5905. https://doi.org/10.1109/ACCESS.2019.2963819
- Sejati, W., Paramitha AH, D., Khansa, F., Maulana, A. S., & Julianingsih, D. (2021). Flood Disaster Mitigation Using the HEC-RAS Application to Determine River Water Levels in the Old City Area of Jakarta. Aptisi Transactions on Technopreneurship (ATT), 4(2), 121–134. https://doi.org/10.34306/att.v4i2.253
- Sharma, K., Anand, D., Sabharwal, M., Tiwari, P. K., Cheikhrouhou, O., & Frikha, T. (2021). A Disaster Management Framework Using Internet of Things-Based Interconnected Devices. Mathematical Problems in Engineering, 2021. https://doi.org/10.1155/2021/9916440
- Susila, M. R. (2021). Model Hybrid Peredaran Uang Elektonik di Indonesia. In STIESIA Surabaya.
- Susila, M. R., Jamil, M., & Santoso, B. H. (2022). Analisis Dampak COVID-19 dan Faktor-Faktor yang Mempengaruhi Indeks Saham Bank Jatim Menggunakan Pendekatan Regresi Time Series. Jambura Journal of Mathematics, 4(2), 220–231.
- Wong, W. M. (2020). Flood Prediction using ARIMA Model in Sungai Melaka, Malaysia. International Journal of Advanced Trends in Computer Science and Engineering, 9(4), 5287–5295. https://doi.org/10.30534/ijatcse/2020/160942020
- Yang, S. N., & Chang, L. C. (2020). Regional inundation forecasting using machine learning techniques with the internet of things. Water (Switzerland), 12(6). https://doi.org/10.3390/W12061578